Reaching out
AI can help landlords identify and assist their most vulnerable tenants
There is lots of talk about how technology can leave some of the most vulnerable service users behind. ‘Digital exclusion’ is a very real concept and one that social landlords – as organisations responsible for the welfare of often ageing and vulnerable customers – have to be acutely aware of. However, AI technology can also be used to assist landlords in their efforts to reach some of their most vulnerable tenants, as Platform Housing Group has discovered.
Silent tenants
Taking its lead from high profile cases of tenants of other social landlords being found dead in their homes having not been contacted for years, Platform’s idea was to use AI and machine learning to improve its service to those most at risk. Using an unsupervised regression-based machine learning algorithm, Platform has been able to identify what it describes as ‘silent tenants’ and place them into a risk category that then dictates how the landlord will engage with them in the future.
“Logistics means we can’t visit every property every two months,” explains Jon Cocker, chief information officer at Platform Housing Group. “So we used a machine learning model with our data scientists to produce the propensity of a customer being silent. We looked at different variables and then intertwined them with our operational and tenancy teams and welfare visits.”
The Midlands-based landlord then used AI tools to help it prioritise visits to those tenants deemed most at risk. Cocker says the AI itself is “continuously updating and learning around what makes a silent tenant”, meaning its identification of those who might be at most risk improves over time.
“There have been some really nice human stories as a direct result of this, where we’ve found people have not contacted us and we’ve insisted that we go and visit them,” continues Cocker. “When we found that they’re living in poor conditions, we’ve managed to get them the help and support they need. So it’s been a really good news story that we’re building on.”
Developing the model
So far, the identification of silent tenants has been restricted to small pilot projects. But as the machine learning model expands out to the entirety of Platform’s 49,000-strong stock, the idea is that it will grow and develop to the point where it is integrated with the organisation’s wider systems.
Explaining how the model works, Platform’s director of data and applications, Rob Fletcher, says: “It looks at huge quantities of data and creates data point clusters. When there’s outlying data points that don’t sit naturally within a cluster, the algorithm then sorts those outliers into high, medium and low risk cases.”
Once these tenants are identified, Platform uses an outbound automated dialler called Voicescape to contact those who are in the outlying high risk data points and asks if they want a tenancy visit.
“If the answer is ‘no’, we remove them from our list, but if there’s no answer, it reinforces the fact they’re a silent tenant,” explains Fletcher. “So if the answer is ‘yes, we would like some support’, or there’s no answer at all, then it triggers targeted tenancy visits.”
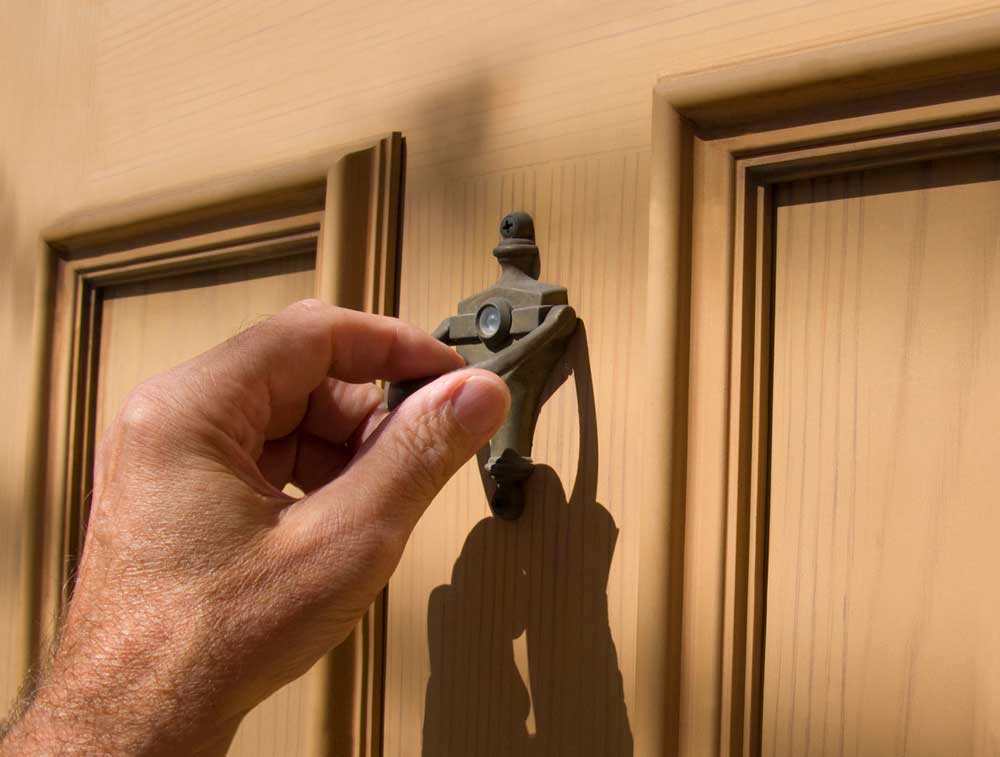
“Logistics means we can’t visit every property every two months.”
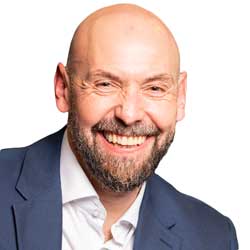
Jon Cocker
Chief Information Officer, Platform Housing Group
“There have been some really nice human stories as a direct result of this, where we’ve found people have not contacted us and we’ve insisted that we go and visit them. When we found that they’re living in poor conditions, we’ve managed to get them the help and support they need.”
Integrating AI
“That’s how it works now, but the future version will enable us to get to a point where we trust those high risk recommendations so confidently that we’re prepared to inject the recommendations straight into the heart of our Customer Relationship Manager (CRM) case management system and remove the human decision-making from the process.
“You’re never going to rule out false positives 100%, but you want to get to a point where you’re 99%-plus confident that you’re not wasting organisational resources because of false positives.”
Integrating AI and machine learning into existing organisational systems is the next step on Platform’s journey, according to Fletcher: “We’ve done a proof of concept where we’re able to infuse our machine learning models within the heart of our CRM and case management platforms. We can genuinely get to a point where we have a discrete number of machine learning models running in real-time, ingesting huge amounts of both our own data and open source external datasets to create predictions – and those predictions can quickly go from predictive to prescriptive.
“So we can inject recommendations from these predictive machine learning models into the CRM, so that cases are created automatically based on the predictions.”
These predictions could be used to prescribe action on everything, from boiler failure to the risk of a tenant falling into rent arrears. And although Fletcher says this model could be deployed this year, it’s likely that Platform will wait until 2025 before moving the project forward.
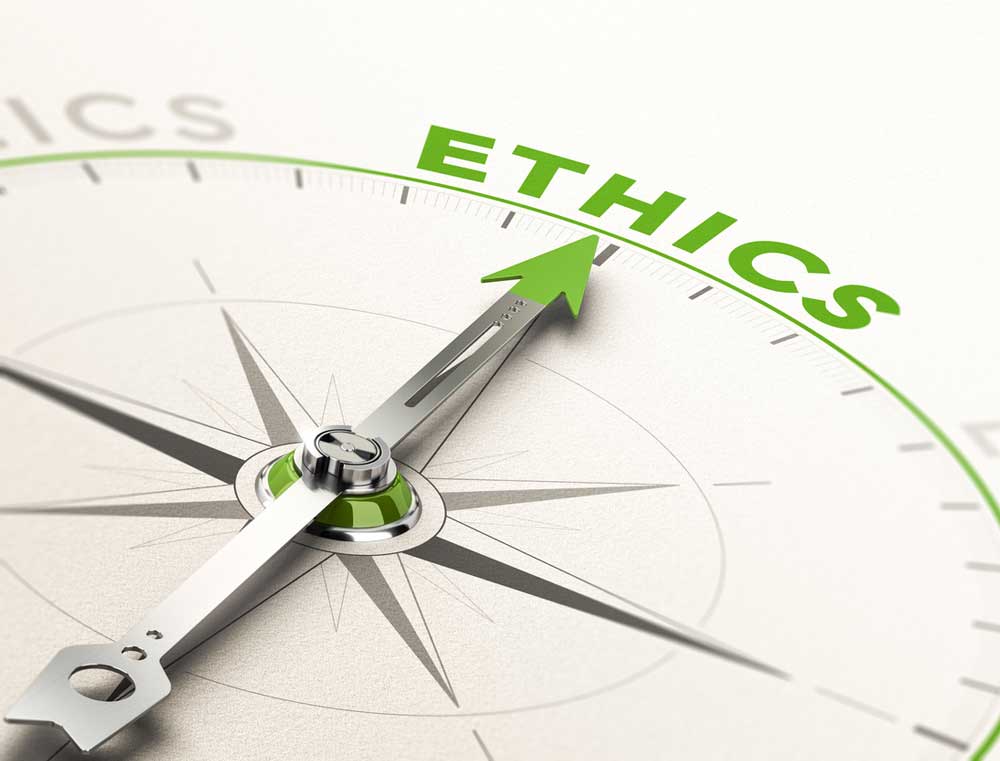
“We have an ethical framework that we developed a couple of years ago that we’ve adapted for AI.”
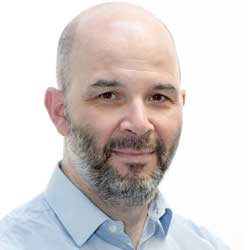
Rob Fletcher
Director of Data and Applications, Platform Housing Group
“We can inject recommendations from these predictive machine learning models into the CRM, so that cases are created automatically based on the predictions.”
Taking things slowly
The delay in rolling out proven technology speaks to another key element of AI for Platform: that it has to be used ethically and with a high degree of tenant buy-in.
“We have an ethical framework that we developed a couple of years ago that we’ve adapted for AI,” says Cocker. “That’s got a number of steps in it, not just to determine what we’re going to do, but whether we should be doing it. There needs to be transparency with the customer about what we’re going to use the information for. And we need to ask ourselves, is artificial intelligence the right tool to do it in the first place?
“It’s that type of question that we need to pick up. That ethical side is a real hot potato because there’s no clear regulation anywhere on what we should be using it for – it can be a bit like the wild west out there at times.”