Biases exist; AI reflects them
When implementing AI technology, organisations have an ethical responsibility to ensure the data used is fair
AI might be set to transform the way housing providers interact with their customers in myriad ways – both known and, as yet, unknown. It is therefore vital that landlords engage with the ethical considerations that come with the adoption of the technology.
Sam Nutt, a researcher and data ethicist at the London Office of Technology and Innovation (LOTI), works with London’s councils on how they can most ethically implement new technology. He says that an awareness of the potential bias inherent in AI should be a key consideration for the housing sector.
Mirror to an unfair world
“We’ve got data that mirrors an unfair world, so I think if you are building things, then even if the data is accurate, it might mirror an historically unfair world, and there’s a chance that you could end up with sometimes quite perverse situations.”
Nutt gives the example of a council looking to reduce how much it pays out to residents in the small claims courts by addressing the concerns or complaints of those most likely to sue. “You might say, ‘let’s look at the profile of the people who sue us for the most money’. You could argue that they will be the people who are most disadvantaged. But it could be a certain profile of people who are more likely to take legal action. Whereas people who maybe don’t have English as a first language or don’t understand the legal system so well, are far less likely to take you to a small claims court and get a payout.
“In that situation, you might think implicitly that the people who sue would be the people who need the most help. But actually, you can end up prioritising helping people who you are only helping because they are better at suing you.
“So your data could be really accurate and the model could be working perfectly, but the model could be working perfectly to reflect an unfair world.”
For Nutt, it’s about being aware of the biases that could be produced by AI models based around, for example, race, gender and sexuality “because they’re trained on unfair data” and coming up with checks and balances to counter them. This is necessarily an iterative process rather than one that seeks perfection.
“Even if you could get it to be technically perfect, if the world is already historically unfair, you could just be continually reproducing that unfair world,” he explains.
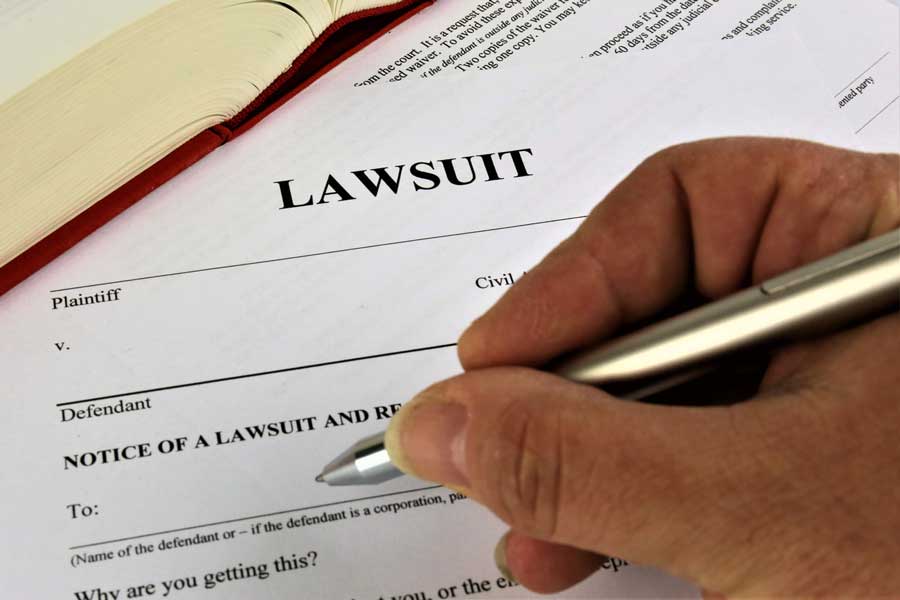
“You might think implicitly that the people who sue would be the people who need the most help. But actually, you can end up prioritising helping people who you are only helping because they are better at suing you.”
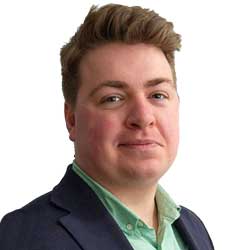
Sam Nutt
Research and Data Ethicist, London Office of Technology and Innovation
“Your data could be really accurate and the model could be working perfectly, but the model could be working perfectly to reflect an unfair world.”
Automating complaints
Applying this principle to a concrete example, Nutt describes how a council he works with is automating responses to some emailed complaints via an AI.
“One of the concerns we had when we were doing this was, what happens if people can write better complaints? Can they game the system? But people can already game the system in that certain types of complaints get better quality responses.” In other words, a bias is likely to exist within a system, irrespective of whether an AI or a human responds.
This use case raises other interesting ethical dilemmas though. One is whether customers and tenants need to know whether their responses are generated by humans or AI.
“When you engage with the council, to what degree are you expecting to speak to a human?” muses Nutt. “One of the things that we talk about at LOTI is the difference between transactional and relational interactions with the public. There are some things that are transactional, and in my view, frankly, humans sometimes get in the way of doing these things effectively. They’re also the tasks that officers don’t particularly want or enjoy or need to do – they’re the tasks we should be looking to use AI for.
“Some complaints cover things that are much more transactional. And in these cases, if you do make that evaluation that there isn’t potential for bias or inaccuracy, or other areas that residents may be particularly concerned with, then I think it’s okay to proceed with them.”
Ethical procurement
A relationship that Nutt views as central to this delicate interplay between landlord and resident, is between those supplying the technology and those who are using it.
“The ethics piece also comes down to the people buying the technology,” he elaborates. “That means procurement and commissioning staff – we need to make sure they are asking the right questions of vendors and suppliers, so that when we’re buying technology, we’re getting the information we need from them in terms of assurance.
“It’s so we know that the models are fair, or that they’re continually being checked for fairness, and they’re going to tell us if something comes up. It’s a big thing for when we’re buying these systems. It’s about doing the assurance really effectively and I think that’s a real priority that we should be thinking about as a sector.”
Assurance is also at the heart of a final point Nutt makes about deploying AI as safely as possible, namely the importance of collaboration. With resources stretched at local government level – just as they are for many housing associations – collaborating to road test new ways of working can create a hugely beneficial shortcut to implementation.
“If you can create safe environments to test these things upstream as early as possible, that would help,” he concludes.” I think it’s a lot of pressure to put on one council to do this for any one thing, which I think is a huge hurdle, so collaboration is important.”
“We need to make sure [procurement and commissioning staff] are asking the right questions of vendors and suppliers.”